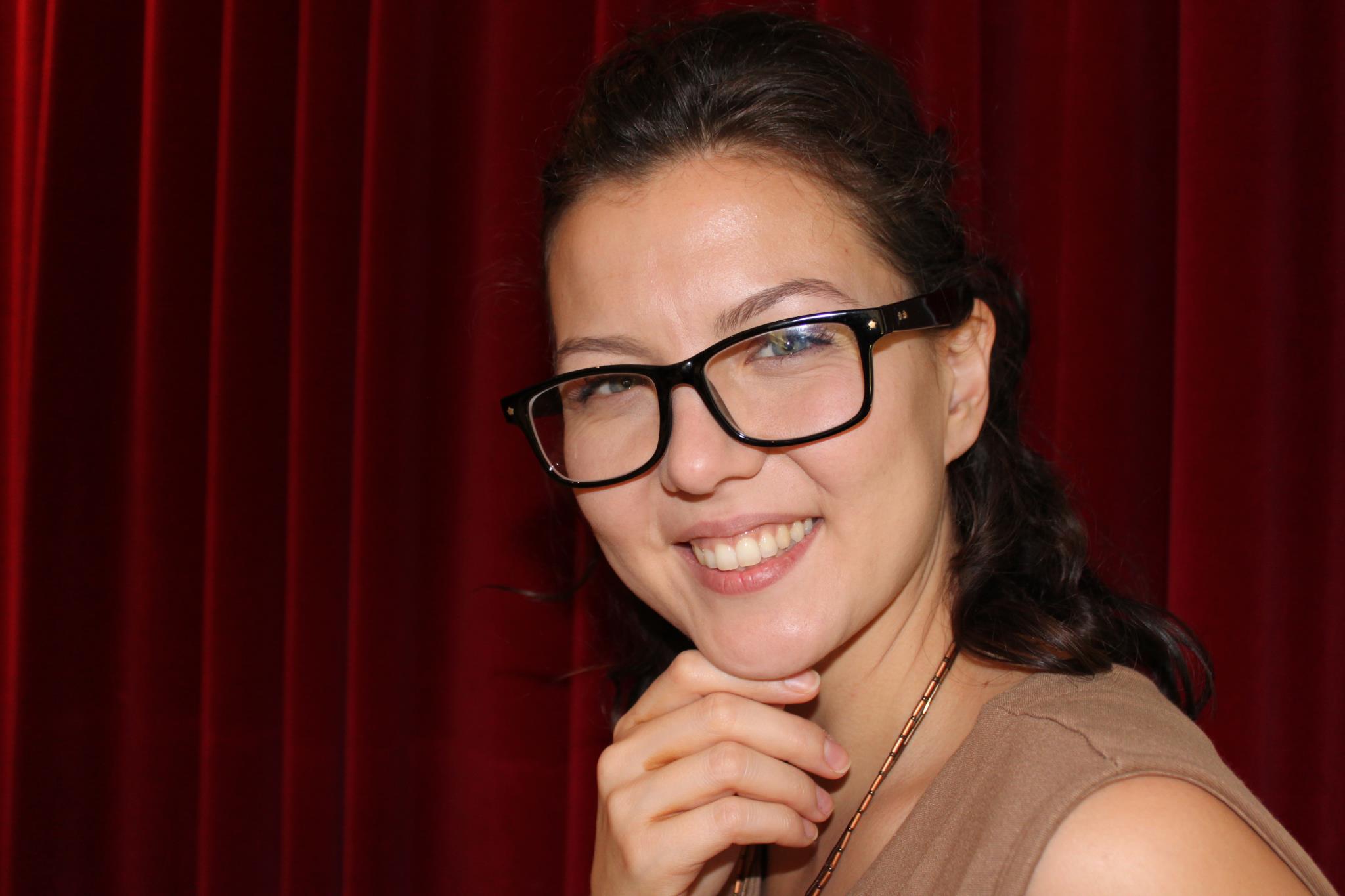
HiringBranch has proven how much better their data set is at creating a language model that can measure soft skills – even next to the nearest best open-source data set! In a recent whitepaper, the team explained how they ran experiments comparing the accuracy of their soft skill language model trained on their proprietary data set versus those that are publicly available. Get a copy of that whitepaper here. To unpack this breakthrough study, we sat down with key HiringBranch linguists and machine learning engineers. Here’s what they had to say.
Interview with HiringBranch Data Scientists
VP of Demand Generation, Chandal Nolasco da Silva, interviewed not one but two HiringBranch data scientists to talk about why the experiments published in their whitepaper were so significant to the skills-based hiring AI economy. Assaf Bar-Moshe, PhD, Chief Research and Development Officer at HiringBranch was joined by Senior NLP and ML Engineer, Vaibhav Kesarwani (aka Vicky), for the conversation.
Chandal: When you set out to run the language model experiments, what problem were you trying to solve?
Assaf: The problem we tried to solve was evaluating people’s soft skills scientifically and automatically so that companies can hire the best talent faster. Our approach was particular. We think of soft skills according to role, and we know that different roles require different soft skills. We had to first define our problem and it was linguistic in nature - how are soft skills expressed with language and what patterns can we understand from human language?
Chandal: And what were your conclusions?
Assaf: The publicly available datasets, or large language models (LLM) are good when you have a general or generic problem. You will find a lot of examples in a big corpus. In our situation, what we needed was specific: a small language model with expertise in soft skills. An LLM turned out not to be a good solution.
What we learned from our experiments was that LLMs do not work well for measuring soft skills, because the data did not have what we needed. It was either insignificant, hidden, or not useful. Our solution was to create a smaller language set with our own proprietary data, and we saw 32-50% more accuracy when the model was trained with it.
Overall there is a lot of hype around LLMs and they are good for general problems, but for specific problems, a dedicated corpus is needed.
Chandal: Talk to me about the size and quality of data sets. What defines a high-quality dataset? How many variables or parameters does our small language model have approximately?
Assaf: The quality of a dataset does rely on the presence of enough data points. You want to see accuracy. However, it has to be the right data for the problem. Despite the fact that large language models typically have billions of data points there still was not enough to solve our exact problem.
Vicky: The parameters vary depending on the language model being tested. So for example, we tested the Google Flan-T5 Base dataset and it has 250 million parameters. Google’s Flan-T5-Large, which was the second most accurate dataset in our experiments, has 780 million parameters. In comparison, HiringBranch’s dataset has 66 million. The Mistral 7B is the largest dataset that was used in the experiment and it has over 7 billion.
“The general rule is that it has to be pre-trained on some generic language tasks to achieve transfer learning and is mostly based on the transformers neural network.”
There is no universal standard that states when a language model is considered large - an LLM. The general rule is that it has to be pre-trained on some generic language tasks to achieve transfer learning and is mostly based on the transformers neural network.
Chandal: What do you think is the biggest takeaway about HiringBranch from your experiments?
“HiringBranch has valuable language, domain knowledge, and datasets that differentiate it from the market.”
Assaf: Smaller datasets can yield more accurate results than larger ones with the right data, and greater accuracy leads to more effective results for our clients. Candidates around the world have taken the HiringBranch assessment through an employer, thus accessing a fairer and more accurate hiring process with our soft skills AI in the process.
What is revolutionary is that we actually did it. After many tiers and trials, improving the system every step of the way, we did what not a lot of other companies have the resources to do. We were resilient enough to solve this unique problem. It wasn’t easy though, and we even went through a process of refining the initial definitions during the experiments. There was definitely an element of continuous learning throughout, and a lot of language model experiments with our own data to get there.
Vicky: Our experiments prove that any random LLM cannot predict soft skills the way we can. HiringBranch has valuable language, domain knowledge, and datasets that differentiate it from the market.
Image Credits
Feature Image: Unsplash/Ilyass SEDDOUG